Love our content? Show your support by following us — pretty please!🥺
FOLLOW ON PINTEREST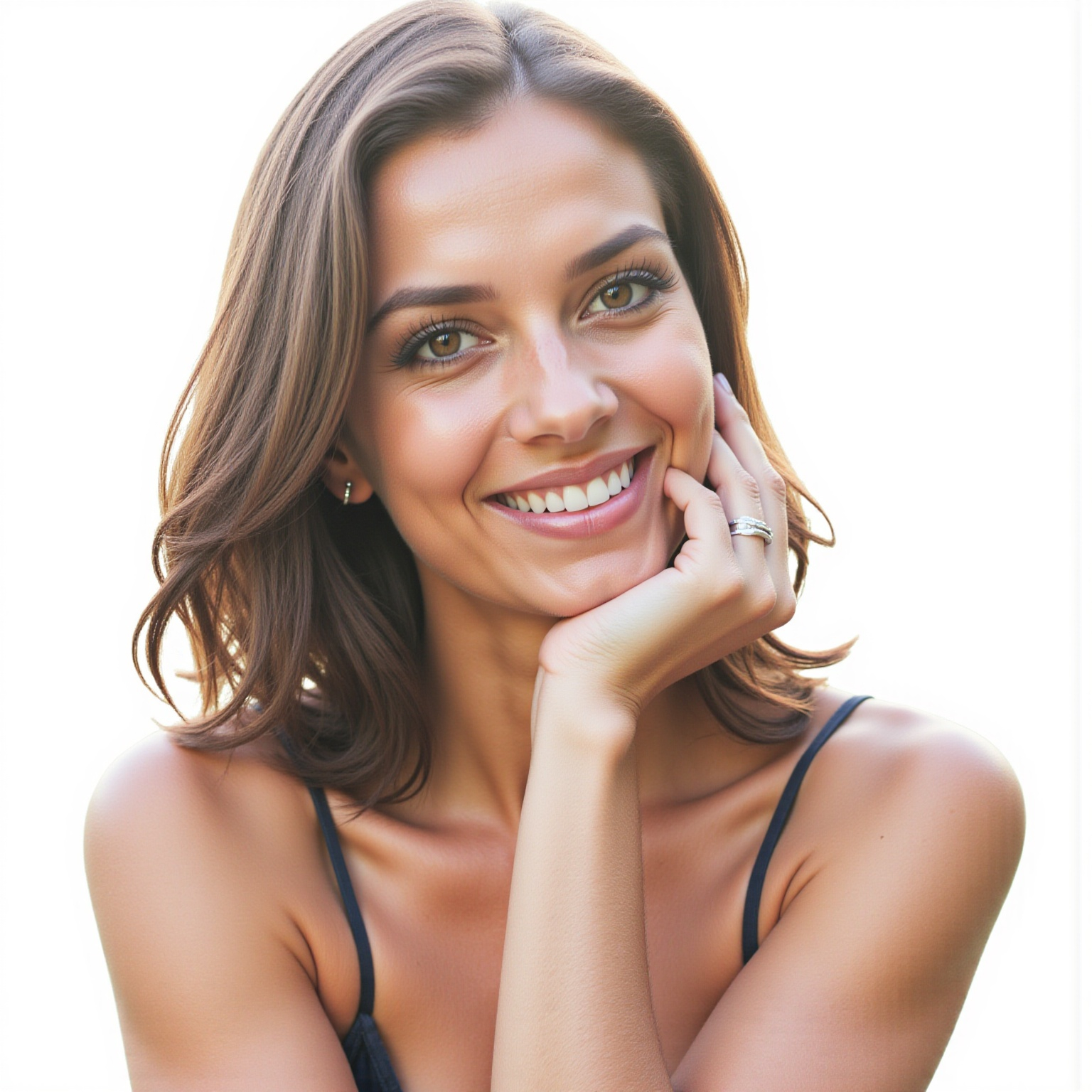
Hi! I’m Kate, the face behind KateFi.com—a blog all about making life easier and more affordable.
For decades, beating the stock market was considered a rare feat reserved for top-tier analysts, fund managers, or those with “inside” knowledge. But we’re in a whole new era—one where artificial intelligence (AI) is transforming the very foundations of investing. From robo-advisors that manage portfolios automatically to hedge funds using machine learning for split-second trades, AI-driven investing strategies are increasingly leaving traditional methods in the dust.
In this comprehensive guide, we’ll pull back the curtain on how these “robot investors” are outperforming some of the most seasoned Wall Street pros. We’ll explore what AI investing really means, how it compares to traditional approaches, and most importantly, how you can tap into these cutting-edge tools. If you’re looking to grow your wealth, diversify your holdings, or simply keep up with one of the biggest revolutions in finance, this guide is for you.
Table of Contents
- The Evolution of AI in Finance
- Why AI Is Beating Traditional Methods
- Types of AI-Powered Investing Tools
- Robo-Advisors Explained
- Algorithmic Trading: From Microseconds to Millions
- Deep Learning, NLP, and Other Advanced Strategies
- AI in Cryptocurrency and Alternative Assets
- Case Studies: Real-World Results
- Risks, Limitations, and Regulatory Concerns
- Practical Steps to Start AI Investing
- Advanced Tactics and Future Outlook
- Conclusion
Extended FAQ
1. The Evolution of AI in Finance
From Ledgers to Algorithms
Investing has come a long way since the days of handwritten ledgers and ticker-tape machines. Traders once relied on delayed market data and personal intuition to make decisions—often to the tune of significant human error. Over the past few decades, computerized systems have taken hold, delivering real-time insights and automating complex tasks. Yet, it wasn’t until the early 2010s that machine learning (a major subfield of AI) really began to reshape the finance world.
Machine Learning Takes Center Stage
Machine learning uses statistical models to detect patterns in data. When applied to stock prices, economic indicators, and company fundamentals, these algorithms learn to predict possible future movements more efficiently than humans. Hedge funds like Renaissance Technologies famously leveraged early machine learning models to earn consistent returns—even during market downturns.
The Democratization of AI
For a long time, AI was perceived as the secret sauce of elite hedge funds. Today, thanks to cloud computing and open-source libraries (like TensorFlow and PyTorch), even retail investors can access robust AI-driven insights. Low-cost robo-advisors, free analytics platforms, and AI-powered brokerage apps are democratizing this once-exclusive technology.
External Link: For an in-depth historical perspective on AI in finance, check out Investopedia’s guide on Algorithmic Trading. It details how algo-trading evolved from basic “rules-based” systems to advanced machine learning strategies.
Internal Link: Want a wider look at how AI is reshaping personal finance beyond stocks? See The Ultimate 2025 Guide to AI in Finance: How to Transform Your Money with Machine Learning.
2. Why AI Is Beating Traditional Methods
Speed and Scalability
Computers excel at ingesting vast datasets in seconds—datasets that would take humans days (or weeks) to analyze. By the time a Wall Street analyst sifts through annual reports and news headlines, an AI might have already processed the data, updated its model, and executed dozens of trades. This speed advantage is crucial in markets where timing is everything.
Data-Driven Objectivity
Human traders are subject to emotional biases—fear, greed, the sunk-cost fallacy, and more. AI systems, on the other hand, rely purely on data and established algorithms. They’re less likely to panic-sell during a dip or buy into hype-driven bubbles without statistical evidence.
Pattern Recognition Superpowers
One of AI’s greatest strengths lies in its ability to detect complex, non-obvious patterns. Whether it’s correlating social media sentiment with earnings surprises or linking commodity prices to small-cap stock performance, advanced machine learning models uncover relationships that even the best analysts might miss.
Adaptability
Financial markets evolve. A strategy that works today may fail tomorrow if a new market regime or economic trend emerges. Adaptive algorithms can recalibrate automatically—often in real time—using updated data to tweak their predictions or trades.
💡 Follow KateFi.com on Pinterest for:
- Frugal living hacks
- Budget-friendly meal ideas
- Creative side hustle tips
- DIY tricks that save you money
External Link: If you want a deep dive into behavioral finance and how biases affect traditional investors, check out NerdWallet’s Behavioral Finance 101 for a look at why emotion-free AI often trumps human intuition.
Internal Link: Learn how these AI-driven insights also apply to everyday budgeting in How to Make Money with ChatGPT: AI-Powered Side Hustles. It’s not just for investing—AI can optimize income streams, too.
3. Types of AI-Powered Investing Tools
When we say “AI investing,” we’re really talking about a range of tools and techniques. Some are more hands-on, while others run on auto-pilot. Here’s a quick rundown:
- Robo-Advisors – Automate portfolio management and rebalancing based on your goals, risk tolerance, and time horizon.
- Algorithmic Trading Platforms – Execute trades (sometimes in microseconds) using AI-driven signals.
- Fundamental Analysis Bots – Scrape company financials, news, and social media sentiment to predict stock performance.
- Market Prediction Models – Provide real-time forecasts of price movements or volatility.
- Portfolio Optimization Tools – Suggest optimal allocations among different assets, from stocks and bonds to crypto and commodities.
Internal Link: Curious about how AI might transform your budget before you invest? Read The AI Budgeting Blueprint: How to Cut Expenses and Save Like a Pro. Reducing unnecessary expenses frees up more capital for your AI-powered investments.
External Link: For a list of popular AI trading and investing platforms, Forbes’ roundup of best robo-advisors can be a handy starting point.
4. Robo-Advisors Explained
The Rise of Automated Portfolio Management
Robo-advisors are online platforms that use algorithms to manage your portfolio automatically. You fill out a questionnaire about your risk tolerance, goals, and time frame, and the robo-advisor constructs a tailored investment strategy—often with minimal fees compared to traditional financial advisors.
How Do They Use AI?
Early robo-advisors relied on simple allocation rules: for example, “80% stocks, 20% bonds” for an aggressive portfolio. Today’s advanced robo-advisors incorporate machine learning models that rebalance portfolios more dynamically. They might reduce stock exposure if they detect rising volatility or shift into safer assets if macro indicators suggest a recession.
Key Benefits
- Low Fees: Robo-advisors typically charge under 0.50% in annual management fees.
- Automatic Rebalancing: No need to manually sell winners or buy losers to maintain allocation.
- Tax-Loss Harvesting: Some robo-advisors use AI to recognize opportunities to sell losing positions to offset capital gains, boosting after-tax returns.
Potential Drawbacks
- Limited Customization: While some allow more advanced tweaking, robo-advisors generally fit you into a model portfolio.
- Lack of Human Guidance: If you value a personal relationship with an advisor, a robo-advisor’s email support might feel lacking.
Internal Link: Looking for real-world examples of how automated tools can transform your finances? Check out I Let AI Manage My Budget for 30 Days — Here’s What Happened!. While focused on budgeting, the experiment also touches on how automation can change money habits drastically.
5. Algorithmic Trading: From Microseconds to Millions
The Essence of Algo-Trading
Algorithmic trading involves using computer programs to execute trades based on predefined conditions. These range from basic “buy low, sell high” triggers to sophisticated AI models that factor in dozens of real-time indicators.
High-Frequency Trading (HFT)
At the extreme end of algo-trading sits high-frequency trading, where machines buy and sell assets within microseconds. Firms race to place their servers physically closer to stock exchanges to shave off tiny latencies. The advantage? Collecting small, consistent gains that accumulate into massive profits over time.
AI in Modern Algorithmic Systems
Traditional algo-trading relied on fixed rules. Modern AI-driven platforms use reinforcement learning to “teach” algorithms which trades are profitable over time. Some incorporate natural language processing (NLP), scanning news headlines or social media sentiment for instant reactions to major announcements (like earnings reports or geopolitical events).
Controversies and Risks
- Market Volatility: Critics argue that HFT can exacerbate flash crashes by executing trades in a split second.
- Regulatory Scrutiny: Governments worry about market manipulation and the lack of transparency in AI’s “black box” decisions.
- Technical Glitches: A single coding error can lead to large-scale losses if the algorithm malfunctions.
External Link: For an authoritative overview of high-frequency trading, the U.S. Securities and Exchange Commission (SEC) guide provides insights into both the benefits and possible pitfalls.
Internal Link: Interested in applying shorter-term trading strategies but also want to keep your finances stable? Explore Stop Living Paycheck to Paycheck: The 21-Day Financial Makeover to ensure you have a stable baseline before diving into riskier trades.
6. Deep Learning, NLP, and Other Advanced Strategies
Deep Learning in Finance
“Deep learning” refers to neural networks with multiple layers that can model complex relationships. In investing, deep learning models might analyze thousands of features—company fundamentals, macroeconomic indicators, even satellite imagery of a retailer’s parking lot—to predict quarterly revenue or stock price movements.
Natural Language Processing (NLP)
NLP is a game-changer for sentiment analysis. Algorithms read through news articles, earnings call transcripts, or even social media posts to gauge investor sentiment about specific companies or sectors. This real-time mood check can signal whether a stock is about to surge or dip.
Reinforcement Learning for Trading Bots
Reinforcement learning is like teaching a dog new tricks—except the “dog” is a trading algorithm. It tries different strategies, receives rewards (profits) or penalties (losses), and iterates until it discovers a pattern that maximizes returns. These dynamic strategies can adapt to market changes faster than a static model.
Hybrid Approaches
Many top-performing AI strategies blend fundamental analysis (e.g., price-to-earnings ratios) with technical signals (e.g., moving averages) and real-time sentiment. The synergy of multiple data streams can produce more robust signals for both short-term trades and long-term holdings.
External Link: If you want to geek out on the tech side, Towards Data Science has comprehensive articles on implementing deep learning and NLP for stock market predictions.
Internal Link: For a more general approach to using AI across different money-related tasks, 7 Easy AI Tools to Turbocharge Your Budgeting This Year offers a good starting point. Even if you’re not coding your own neural network, these tools can significantly optimize your finances.
7. AI in Cryptocurrency and Alternative Assets
Beyond Stocks and Bonds
AI’s advantages aren’t limited to traditional markets. In the volatile world of cryptocurrency, where prices can swing by double digits in a single day, AI-based bots can adapt faster than human traders, seizing opportunities (or averting risks) instantly.
Evaluating Crypto Projects
Machine learning models also help cut through crypto hype. By examining on-chain data—like transaction volumes and network adoption—plus external signals like social media sentiment, AI tools can predict whether a coin is being pumped artificially or genuinely gaining traction.
Other Alternative Assets
From real estate crowdfunding platforms to commodities (oil, gold, wheat) and even NFTs, AI can help parse massive streams of data to find undervalued assets. It might track weather patterns for agricultural commodities or monitor real estate listings to spot local market imbalances.
Internal Link: Thinking about investing in crypto but still building up your capital? See How to Make Money with ChatGPT: AI-Powered Side Hustles for ways to generate extra income streams you can funnel into alternative assets.
External Link: For more insights on AI-based cryptocurrency trading, CoinDesk’s guides are a solid starting point. They often discuss how major crypto funds leverage advanced algorithms.
8. Case Studies: Real-World Results
Renaissance Technologies
No conversation about AI in finance would be complete without mentioning Renaissance Technologies’ Medallion Fund. Led by mathematicians rather than traditional finance pros, it has returned an average annual gain of ~66% (before fees) since 1988. Their secret? Advanced statistical and AI models.
Bridgewater Associates
Ray Dalio’s hedge fund invests billions using systematic strategies, including machine learning. While not purely AI-driven, Bridgewater employs data-driven methods to remove emotional biases. Its flagship fund has consistently outperformed many traditional managers.
Individual Investors’ Success
It’s not just billionaire hedge funds benefiting. Plenty of retail investors share stories on forums like Reddit’s r/algotrading about how their AI-based signals led to successful trades. While less flashy than the big players, these anecdotes highlight the accessibility of AI tools.
Robo-Advisor Outcomes
Firms like Betterment, Wealthfront, and M1 Finance have gained popularity. Many users report stable, consistent growth in line with (or sometimes above) comparable index funds, largely because of intelligent rebalancing and cost-effective operations.
External Link: Bloomberg often reports on AI-based hedge fund performance. You can find articles on how top funds implement machine learning and compare results to standard benchmarks.
Internal Link: If these case studies inspire you to adopt an AI-first approach to your entire financial life, see From Debt to Freedom: How AI Can Automate Your Debt Payoff. It shows how the same principles apply to tackling personal debt efficiently.
9. Risks, Limitations, and Regulatory Concerns
Overfitting and Model Risk
One of the biggest pitfalls in machine learning is overfitting—training your model so specifically on historical data that it fails to generalize to new market conditions. A strategy that excelled from 2016 to 2020 might crash in 2023 if the market regime changes.
Data Quality
AI is only as good as the data it feeds on. If the data is incomplete, biased, or inaccurate, the model’s decisions can be flawed. This risk is amplified in alternative asset classes or emerging markets where reliable data is scarce.
Regulatory Hurdles
As AI-driven trading intensifies, regulators (like the SEC) are keeping a close eye. The fear: these black-box models might unintentionally (or intentionally) manipulate markets. Expect more oversight and compliance measures as AI’s presence grows.
Ethical Considerations
Can an AI cause a “flash crash” or engage in predatory trading without human oversight? Possibly. Large-scale AI adoption raises questions of fairness, market stability, and accountability.
External Link: The Financial Industry Regulatory Authority (FINRA) occasionally publishes reports on automated trading compliance. Check there for guidelines if you’re considering a more advanced AI trading approach.
Internal Link: Need a safety net in case your AI-driven trades go south? Your Financial Safety Net: Building Emergency Funds Without the Stress teaches how to maintain a robust cushion while venturing into riskier investments.
10. Practical Steps to Start AI Investing
- Assess Your Goals
Are you aiming for long-term growth via a robo-advisor or short-term algorithmic trading? Clarifying your objectives guides your choice of AI tools. - Research Platforms
- Robo-Advisors (e.g., Betterment, Wealthfront) for passive investors.
- Social Trading Platforms (e.g., eToro) with copy-trading features.
- DIY AI Tools (e.g., QuantConnect, Kaggle) if you prefer building your own models.
- Start Small
Experiment with a modest amount of capital. Evaluate performance over a few months to see if the results align with your risk tolerance. - Diversify
No matter how advanced the AI, remember the adage: “Don’t put all your eggs in one basket.” Spread your money across multiple strategies or asset classes. - Monitor and Adjust
While AI handles many decisions, you still need periodic check-ins. Market conditions change, and so might your personal goals. - Stay Updated
Follow finance and AI news to remain aware of new regulations, emerging tools, or changes in your chosen platform’s performance.
Internal Link: For more ways to free up investment capital, see Side Hustles That Work in 2025: The Best Ways to Earn an Extra $2,000 a Month.
External Link: If you’re leaning towards building or customizing your own algorithms, QuantConnect’s open-source platform offers tutorials and a community of DIY quants.
11. Advanced Tactics and Future Outlook
Quantum Computing Meets AI
It might sound sci-fi, but quantum computers are on the horizon. In theory, they can handle computations exponentially faster than conventional computers, enabling even more sophisticated AI models. Hedge funds are already preparing for the day quantum-based AI can decode complex market signals overnight.
ESG and Thematic AI Strategies
Ethical investing is a hot trend. Expect to see AI-based ESG (Environmental, Social, Governance) scoring systems that sift through corporate actions and supply chain data to find truly sustainable or socially responsible investments.
Fractional Share Investing with AI
Small retail investors can now buy fractional shares of high-priced stocks. AI can optimize how many fractional shares you should hold to maintain your ideal asset allocation or exploit short-term price dislocations.
Potential for Full Automation
Imagine a future where your finances—budget, investments, insurance—are all seamlessly managed by interconnected AI services. You’d simply set overall life goals (like retirement age or a dream home purchase), and the “machine” would orchestrate every financial move to meet these milestones efficiently.
External Link: World Economic Forum has several articles forecasting how AI will transform global finance over the next decade.
Internal Link: If your goal is early retirement, check out Financial Independence, Retire Early (FIRE): A Comprehensive Blueprint. Incorporating AI investing could accelerate your path to financial freedom.
12. Conclusion
The days when only elite hedge funds wielded AI for eye-popping returns are over. AI-driven investing tools—from robo-advisors to advanced quant strategies—are increasingly accessible to anyone with an internet connection and a willingness to learn. While no system (human or machine) guarantees perpetual profits, AI’s speed, adaptability, and data-driven objectivity can offer a distinct edge in a complex marketplace.
Whether you’re a novice building a long-term portfolio or a trader chasing short-term alpha, AI provides resources that can simplify decision-making and potentially amplify returns. The key is balancing risk management, diversification, and ongoing due diligence. After all, the ultimate power of AI emerges when combined with human oversight—letting you ride the wave of innovation while steering clear of pitfalls.
So if you’ve been eyeing the success of AI-based hedge funds and longing for a piece of that automated magic, there’s never been a better time to get started. Do your research, pick a platform, start small, and watch how the synergy between human intelligence and artificial intelligence can reshape your financial future.
Extended FAQ
Q: Can AI guarantee outperformance over the long run?
A: No. Markets evolve, and even advanced algorithms can fail if they don’t adapt or if market conditions shift drastically. However, many AI-driven tools have historically beaten traditional benchmarks under certain conditions.
Q: Will AI replace human fund managers entirely?
A: AI has replaced many tasks, but human oversight is still crucial. Markets are driven by macroeconomic events, politics, and human sentiment—factors that might require emotional intelligence or ethical judgment AI doesn’t possess (yet).
Q: Is algorithmic trading too risky for retail investors?
A: It can be. High-frequency strategies often require fast connections, specialized knowledge, and a big bankroll to be competitive. But simpler AI-based methods—like robo-advisors—are designed with risk management in mind.
Q: How much money do I need to start with a robo-advisor?
A: Some have no minimum deposit; others might require $500 or $1,000. It varies by platform. The important part is your time horizon and risk tolerance.
Q: Do I need coding skills to benefit from AI investing?
A: Not necessarily. Many platforms handle the technical side. If you want to build custom strategies or implement sophisticated trading algorithms, coding knowledge helps. But mainstream robo-advisors and algorithmic services handle everything behind the scenes.
Q: What if I’m more interested in stable, dividend-paying stocks than short-term trading?
A: AI can help there too, analyzing dividend histories, payout ratios, and sector trends to identify consistent dividend payers. You can combine AI insights with your personal preference for steady income.
Q: Where can I learn more about the ethical considerations of AI in finance?
A: Regulatory bodies like the SEC and FINRA often publish guidelines. Many universities also explore AI ethics in finance. As AI becomes more prevalent, expect more discourse on accountability and fairness in algorithmic decisions.
AI investing isn’t a passing fad—it’s reshaping the very fabric of capital markets. From lightning-fast algorithmic trades to robo-advisors that simplify money management, the era of human-only strategies is giving way to a powerful partnership of human-plus-machine. Will you sit on the sidelines or embrace the future? The choice, and potential rewards, are yours.